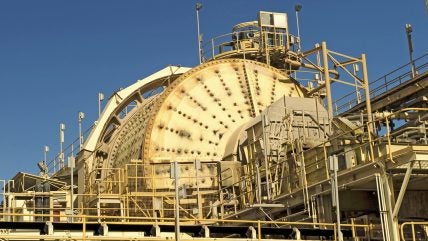
Crushing and grinding are integral processes in mineral extraction, but like a lot of areas of the mining industry, they have traditionally relied heavily on human expertise and manual operation, which carries with it all the risk of human error.
Enter AI-powered systems, equipped with advanced algorithms and machine learning (ML) capabilities. These systems can analyse vast amounts of data in real time to maximise efficiency and product quality. By continuously learning from operational data and adjusting systems accordingly, AI-driven crushing and grinding systems can achieve unprecedented levels of precision and performance – but how is the industry putting the tech to use, and is it all it is cracked up to be?
The energy problem
One of the most pressing issues in crushing and grinding is the sheer amount of energy these processes use. They account for around 56% of the mining sector’s total energy usage – which adds up to 3% of the entire planet’s generated electric power usage. Given that one of the major factors driving the push for greater mining output at present is the increasingly urgent need for electrification and critical minerals to power it, reducing energy consumption within mining processes is hugely important.
This is one area where AI has already shown major promise for crushing and grinding. AI algorithms can help save energy and cut emissions by tweaking equipment settings to fit different operating situations. This means that AI-powered crushing and grinding systems don’t just crush rocks – they also help mining companies be more environmentally responsible by using resources more efficiently, which is important as the industry pays more attention to the environment.
An October 2023 paper by researchers at the University of Arizona, Tucson, published in the journal Minerals, has demonstrated significant potential for the implementation of ML algorithms into crushing and grinding processes.
The researchers examined the efficiency of SAG mills and found that machine learning algorithms were successfully able to identify and predict particle cluster size during the grinding process.
How well do you really know your competitors?
Access the most comprehensive Company Profiles on the market, powered by GlobalData. Save hours of research. Gain competitive edge.
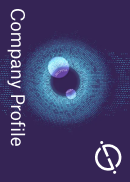
Thank you!
Your download email will arrive shortly
Not ready to buy yet? Download a free sample
We are confident about the unique quality of our Company Profiles. However, we want you to make the most beneficial decision for your business, so we offer a free sample that you can download by submitting the below form
By GlobalDataThe study’s authors are extremely optimistic about the potential impact this could have on energy efficiency in crushing and grinding processes, stating that “these results are suitable to be integrated into real-time mill control systems, thus providing control designers and operators with tools to optimize performance that can lead to ensuring the necessary production rate while achieving energy optimization”.
Keeping on that grind: predictive maintenance
Maintenance of crushing and grinding equipment is crucial for minimising downtime and maximising productivity. However, maintenance practices are often reactive rather than proactive or predictive, leading to unexpected breakdowns, production stoppages and costly repairs.
AI-enabled predictive maintenance solutions are changing the game by monitoring equipment performance in real time and predicting potential failures before they occur. By analysing data from sensors and spotting patterns that signal trouble ahead, these systems save time and money.
AI algorithms can also help by adjusting equipment settings and adapting how machinery runs based on real-time conditions; this means that more gets done while using less energy and putting less strain on the machinery. It is well known that taking a proactive attitude to asset management not only enhances operational efficiency but also extends the lifespan of equipment, delivering significant cost savings over time, and AI is proving to be a useful tool in this endeavour.
While hard data on exactly how much more efficient crushing and grinding can become when AI-powered predictive maintenance tools are used is still limited, it is widely agreed that sensor and AI-based predictive maintenance has a significant positive impact.
Methodologies for incorporating this technology are numerous and varied. One approach currently in development is laid out in a 2022 paper by a team of Moroccan researchers, who hope to further test their methodology in real-world environments in the coming months.
How AI can boost crushing and grinding accuracy
Crushing and grinding processes need to produce consistent and accurate output at all times. Over-processing leads to poor recovery rates and yields, and wasted materials, while under-processed material may need to be reprocessed, wasting time and energy and further wearing down machinery.
Furthermore, if machinery is overloaded, excess material could cause significant damage to the system, and variations in the density of materials may make it challenging to identify at a glance just how much material is too much.
Here, AI is also proving useful, and the inspiration for this development comes from a surprising source: video games.
A study conducted by Boliden in collaboration with ABB used algorithms adapted from video games to try and develop an ML model that could consistently identify the optimal ore load size for crushing machinery. As Boliden development engineer and project manager Johannes Sikström explains in a Boliden blog post, the nature of video games makes their code ideal for adapting into ML algorithms for many different uses.
“In the case of self-learning algorithms – so-called deep learning or reinforcement learning – the challenge is the great quantity of data necessary for the algorithm to learn enough about the system for it to make effective decisions,” Sikström says. “This is why games are such a major area within AI research. Games are well suited to enable algorithms to train themselves, and what constitutes a successful result – a win – is also well defined.”
In this project, the researchers developed and tested an algorithm to control the load size entering grinding machinery to within a certain range. “We directed our attention to the grinding process in Aitik, where we have a well-developed simulation model,” Sikström says. “We wanted to see if AI was able to do better than our existing control strategy. We were then able to test various algorithms and different goal functions.”
In around 80 hours, the algorithm had taught itself to run the machinery in such a way as to produce maximum profitability. It provides an exciting use case for AI technology in the grinding process, showing just how quickly positive changes can be implemented, but as Sikström notes, having knowledgeable people on hand to help develop these systems will always be critically important.
“Because an algorithm is only able to solve the problems formulated for it, process know-how and experience are at least as important in this type of development as classic process control,” he adds.
Leveraging AI’s potential in mining operations
As the mining industry continues to embrace digital transformation, the role of AI in crushing and grinding processes will only become more significant. With ongoing advancements in AI technologies and data analytics, mining companies have never had greater opportunities to boost efficiency and ensure the sustainability of their operations.
However, tapping into AI’s full power in mining calls for teamwork among industry players, tech companies and regulators. By nurturing innovation and backing AI-driven solutions, the mining sector can reach higher levels of productivity, profitability and environmental responsibility, leading us towards a future of mining that is both efficient and responsible.