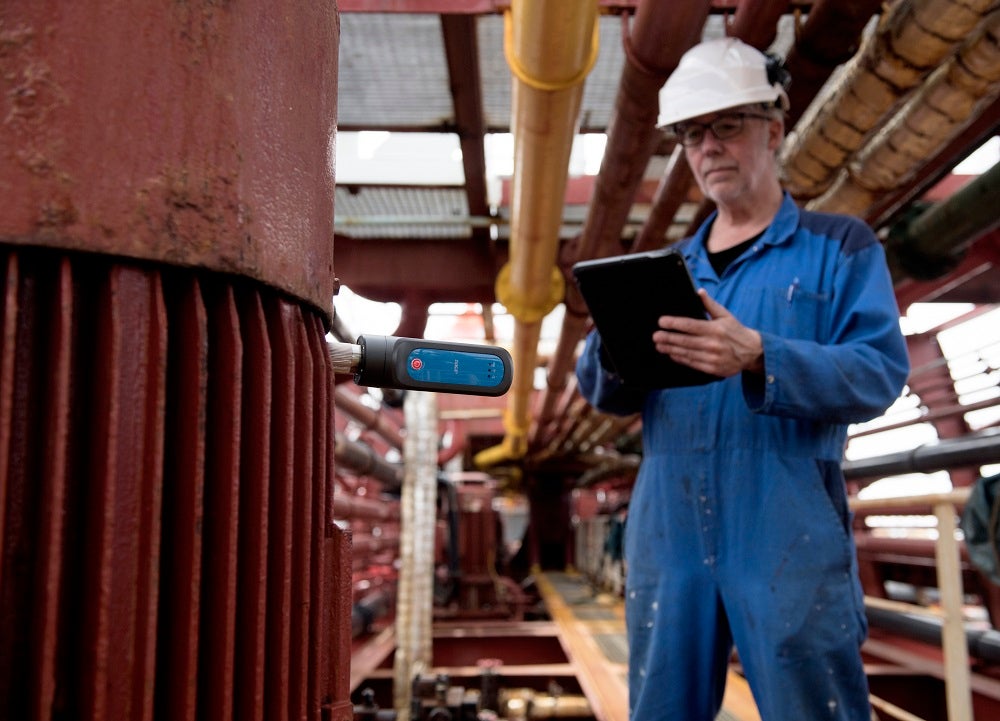
SKF research gives customers new tools in the war against unplanned downtime, says Bernie Van Leeuwen, Head of SKF’s Research & Technology Development.
Gothenburg, Sweden 7 October 2020: Customers come to SKF because they want products and services that support their reliability, sustainability and performance goals. The overwhelming majority of the time, that’s exactly what they get. More than 90% of bearings last longer than the machines in which they are installed.
In our R&D work, we spend a lot of our time thinking about the small minority of products that do eventually fail in service. Over the years, we have developed sophisticated models that can determine how long any bearing will run under known operating conditions before its reliability is compromised by accumulated fatigue.
The real world isn’t like the lab, however. By investigating issues in the field, and by analysing bearings that come back from customers, we know that nine out of ten in-service failures happen when conditions deviate from the expected: problems with lubrication, contamination, corrosion or damage from stray electrical currents, for example.
Machines need to work in tough conditions as well, so issues like these are never going to go away. But if we can get better at predicting these relatively rare events, we can reduce their disruptive impact. Replacing a bearing during a scheduled overhaul is far better and less costly than stopping an expensive production line for emergency repairs.
Today, SKF is involved in a wide-ranging effort to improve our predictive power. Our work aims to answer three basic questions for customers:
- Detection. Is there an issue, or is there likely to be an issue, with my machine?
- Diagnosis. What is the underlying root cause of the issue?
- Prognosis. Can I continue to run my machine until its next scheduled maintenance interval? Can I take any action now to preserve its health?
Our efforts to answer those questions have led us into new areas of technology. They have also required us to apply well-established approaches in new ways.
Take bearing testing for example. We operate dozens of bearing test rigs in our labs around the world. Their job is to run our products to destruction, helping us understand failure mechanisms and verify our mathematical models. The traditional way to do such tests is quite simple. You run the rig until you detect a problem with the bearing, then you stop.
For our predictability work, we need a different type of test, one that starts when a conventional test would stop. We want to understand how damage progresses in a bearing and how rapidly small defects develop into major problems. That knowledge will help customers to understand how long they can continue to operate if the sensors on their machines detect a defect.
To facilitate this new type of testing, we are building a dedicated facility at our Research and Technology Development (RTD) centre in Houten, The Netherlands. Called Bearing Rigs for Accelerated Verification Experiments (BRAVE), the equipment in the new centre is designed to replicate the kinds of “imperfect” conditions you find in the real world. We have special rigs designed to generate defects in bearings, for example, by corroding them, starving them of lubrication, or exposing them to dirt or electric currents. Then we have other rigs that allow us to see how different loads and speeds influence the rate at which the damage spreads.
Another area where we are doing a lot of work is in the application of artificial intelligence and machine learning. Over the past year, we have greatly increased the size and strength of our SKF AI group, and now we are adapting systems and processes across the organisation to make better use of those capabilities.
Our latest test rigs, for example, collect more data at higher rates than their predecessors, and we store that data in the SKF cloud, so teams around the world can access it to train their algorithms and test new ideas.
We are also applying automated analysis to new types of data. A really good example of that is an ongoing project with our remanufacturing business. Steel mills use lots of large bearings in their materials handling systems. Their size and cost make them suitable for remanufacturing.
If a bearing with a fault has been running for too long, the severity of its damage can mean that it can not be remanufactured. Until now, the problem has been that we have not known if a bearing is suitable for remanufacture until it arrives at one of our processing facilities. To avoid unnecessary transport, we are training an automated image recognition system. Customers will take photographs of bearings when they dismount them, and the system will evaluate its condition to see whether it is suitable for remanufacture or needs to be scrapped. We hope the system will also offer extra value for customers, providing them with a report that outlines the likely root-cause of the damage in their bearings.
One of the most exciting things about working with big data and AI is the way the different strands reinforce each other. Combining data from our new test rigs, from sensors installed on customer machines, and even from images of damaged bearings, helps us to continually refine and improve our algorithms and mathematical models.
We have already made some significant breakthroughs, especially in the areas of detection and diagnosis, and we are using those techniques with specific customers today. As our methods become more robust and more widely applicable, we plan to roll out a number of new tools, products and service offerings in the coming months and years.