
Crushing and grinding remains one of the most energy-intensive processes in mining, often accounting for almost half of a mining operation’s overall power usage – and potentially up to 1% of the entire planet’s generated electric power usage.
The process is an integral part of almost all extractions, reducing the size of ore to better retrieve valuable minerals and preparing it for further processing. However, like many areas of the mining industry, crushing and grinding has traditionally relied on human expertise and manual operation, which carries with it the risk of human error.
However, with repairs, inefficiencies and a wide array of ore types all having to be dealt with, Mining Technology looks at whether AI – equipped with advanced algorithms capable of analysing vast amounts of data in real time – will play a bigger part in crushing and grinding operations as the industry looks to become more efficient, reduce energy usage and cut carbon emissions.
The challenges of crushing and grinding
“Crushing and grinding, sometimes called comminution, are among the most energy intensive processes in mining, accounting for up to 50% of a mine’s total energy consumption,” say Wilson Monteiro, global business line manager, and Maarten van de Vijfeijken, grinding product marketing manager, at ABB Process Industries.
“However, high energy cost is just one of the challenges of the process, with equipment wear and tear, operational inefficiencies and variability in ore properties, including declining ore grades, adding additional strain to the journey to more sustainable, efficient grinding operations,” they add.
For example, hard or abrasive ores can cause rapid wear and tear on crushers and grinding mills, with regular monitoring and part replacement helping to minimise wear damage (and the inevitable associated downtime).
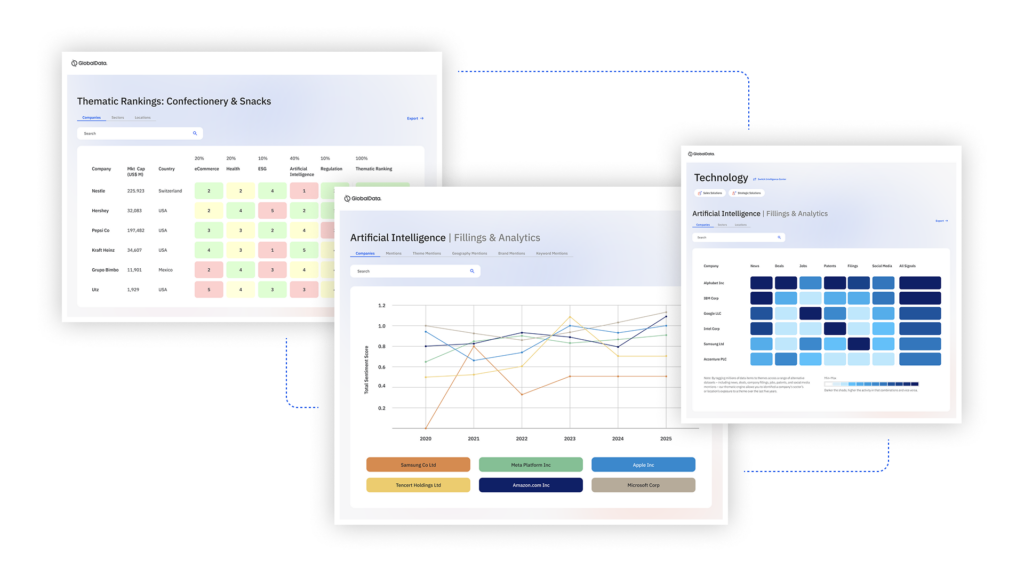
US Tariffs are shifting - will you react or anticipate?
Don’t let policy changes catch you off guard. Stay proactive with real-time data and expert analysis.
By GlobalData“Harder ores require more energy to break, while variations in ore properties can lead to fluctuations in process performance,” the pair add. Additionally, “lower ore grades typically require larger throughputs and consequently larger process equipment, making it essential to use economies of scale to process low-grade ore economically”.
How AI is improving crushing and grinding
There is “no one-size-fits-all solution when it comes to enhancing the efficiency of crushing and grinding operations, but AI is an important piece of the puzzle”, according to ABB. The company points specifically to the potential of AI-driven process control and predictive maintenance capabilities.
Machine learning (ML) models are able to “analyse a flood of real-time sensor data to predict equipment wear, optimise mill loading and adjust operating parameters dynamically”, say Monteiro and van de Vijfeijken.
AI-driven process control systems can reduce energy consumption, improve throughput and enhance ore recovery.
“AI can help to keep [crushing and grinding] circuits operating optimally to minimise recovery loss and maximise overall process efficiency,” the pair add. For example, AI-powered digital twins that simulate grinding circuits can enable mines to test different operating scenarios before implementing changes in real time.
Using AI to power predictive maintenance solutions also minimises unplanned downtime by identifying potential equipment failures before they occur. The ABB experts argue that while AI is “not a stand-alone solution, its integration with advanced automation and data analytics significantly improves the efficiency and cost-effectiveness of comminution processes”.
Monteiro points to the benefits of the gearless mill drive (GMD), which “inherently boosts mill availability by eliminating all mechanical drive train components and generates a constant stream of data that can be analysed by AI tools to detect unusual patterns and trends early on”.
In mid-March, ABB introduced GMD Copilot, an AI-powered digital assistant designed to optimise the operation and maintenance of GMDs. The company also claims that by eliminating “all mechanical drivetrain components and being able to operate at large scale, GMDs deliver more efficient, reliable operations with lower emissions”.
For Grant McHenry, Asia-Pacific regional commercial manager, Rockwell Automation, technology is now offering ways to achieve increases in throughput and yield while reducing specific energy use. This can be achieved with established advanced process control (APC) and newer technologies such as digital twins and AI.
However, when asked if AI is the best way to enhance the efficiency of crushing and grinding in the mining industry, McHenry replied: “Not necessarily, and in fact we found that the best approach is to first understand the problem the miner has and then look for the right technology and engineering services to solve the problem.
“AI is certainly in the mix, but jumping to the latest shiny thing may not actually solve the problem,” he adds.
Advanced process control techniques
One way to “better understand techniques to improve the process performance” is through “process optimisation, using data analytics in the assessment phase of a crushing or grinding application”, McHenry notes.
Then, depending on the problem, the recommended solutions can “apply very mature technologies such as model predictive control [MPC]… [or] new technologies such as AI”, he says.
“Solutions to these problems are often technical in nature, and we have applied our Pavilion8 Model Predictive Control technology to a number of crushing and grinding circuits in recent years to great effect,” says McHenry. “Each time we are targeting either an increase in throughput, an increase in yield, a reduction of specific energy, a reduction of wear and tear, increased consistency of grind and a reduced reliance on operator intervention.”
MPC is a type of advanced process control technology. Advanced process control systems traditionally use linear or non-linear mathematical models of the industrial plant alongside smart algorithms to estimate unmeasured states and control process variables. The systems are used to help optimise processes by predicting future behaviours.
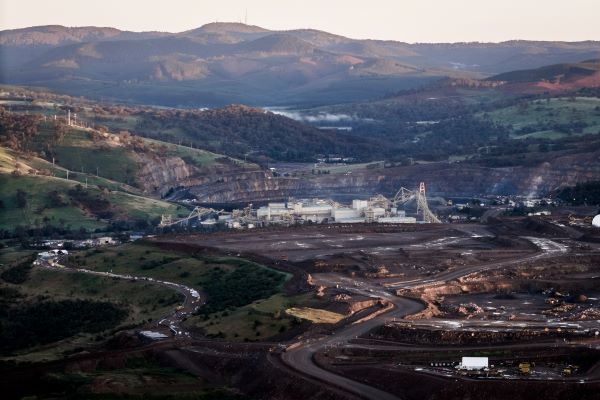
Several years ago, Newcrest Mining, one of Australia’s leading gold miners and a major stakeholder at the Cadia facility in New South Wales, needed to increase the stability of the overall process for recovering gold from ore.
According to a Rockwell case study, Newcrest commissioned the assessment of 12 months of historical data to identify the key process parameters that could be controlled and modified. Pavilion8 MPC was used to predict how the process would respond to variability and outside disturbances, then adjust for desired results.
Rockwell found that to maximise throughput, the grinding circuit needed to be run as quickly and efficiently as possible against downstream constraints: grinding incoming ore down to appropriately sized particles in as few repetitions of the circuit as possible. The MPC controller monitored and adjusted for multiple variables simultaneously, including semi-autogenous grinding (SAG) mill speed, rate of ore feed and water flow into the mill, among others.
ABB also states that the advanced process control systems optimise grinding circuits by continuously adjusting parameters based on real-time data while sensor technologies help improve ore processing by providing detailed insights into mill performance.
Enhancing crushing and griding operations
There are several non-AI techniques that can be used to enhance crushing and grinding operations.
“Stripping back technical innovations and AI advancements, operational best practices and sustainable mine planning can significantly improve comminution efficiency,” says van de Vijfeijken.
For example, ore pre-concentration techniques such as sensor-based sorting can remove waste material before grinding. Blasting optimisation enhances fragmentation, “ensuring that the ore entering crushers and mills is of an optimal size, both of which result in reduced energy requirements and consumption”, van de Vijfeijken adds.
“New product development and the use of simulation tools like discrete element modelling also contribute to better efficiency. This is because they can customise the liners and processes to the mine’s specific ore body,” Mark Hurley, executive general manager at Bradken, a subsidiary of Hitachi Construction Machinery specialising in wear solutions for mining equipment, tells Mining Technology.
“This customisation – matching the wear liners to the ore body – can increase throughput, improve the life of the mill and liners, and it can also achieve the best grind for recovery.”
While there are several traditional approaches for optimising crushing and grinding, AI and ML tools are expected to play an increasing role in future.
For example, APC systems traditionally operate independently from AI; however, the rise in AI is leading to integration between the technologies, according to ABB.
H-E Parts International, part of HCM, which offers crusher maintenance services and consumables, also sees a role for ML in enhancing crushing and grinding operations.
“ML is key for analysing large datasets, detecting patterns, predicting outcomes and optimising process parameters for greater efficiency. This is where HEPI firmly believes the future of asset optimisation will stem from,” it says.
However, despite the growing potential of AI, it is not always the go-to solution for optimising crushing and grinding operations.
“There is always more than one way to solve a problem,” McHenry concludes, emphasising that it is important to identify what technological solution would be best fit for a particular problem.
“It all comes down to what the problem is and the miners’ business driver: is it throughput? Is it yield? Is it energy savings?” Depending on the question, businesses should find their AI or non-AI answer.